Hello folks, IOblend here. Hope you are all keeping well.
There is one thing that has been bugging us recently, which led to the writing of this blog. While working on several data projects with some of our clients, we observed instances when data lineage had not been implemented as part of the solutions. In a couple of cases, data lineage was entirely overlooked, which raised our eyebrows.
Data lineage is paramount from the data auditing point of view. How else would you keep track of what is happening to your data throughout its lifecycle? What if your systems go down and the data becomes corrupted? How would you know what data generated spurious results down the line? You will really struggle to restore your data to the correct state if you do not know where the problem is.
The common reason for data lineage omission was the time pressure to deploy a new system. Delivering the system was considered a much higher priority than ensuring the data quality that fed it. We get it, designing and scripting data lineage across your entire dataflows and data estate can be a massive undertaking, especially under time and resource pressure.
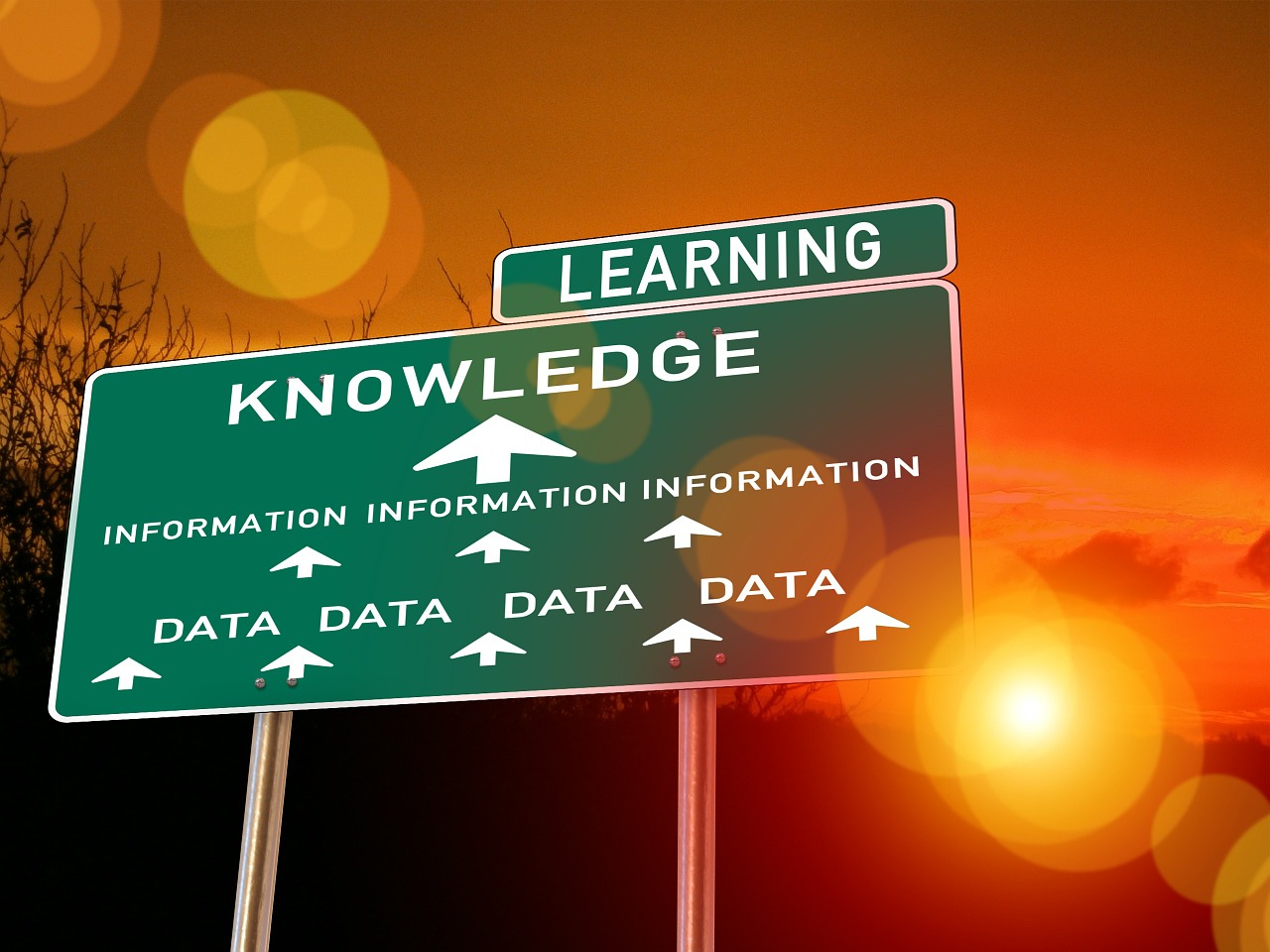
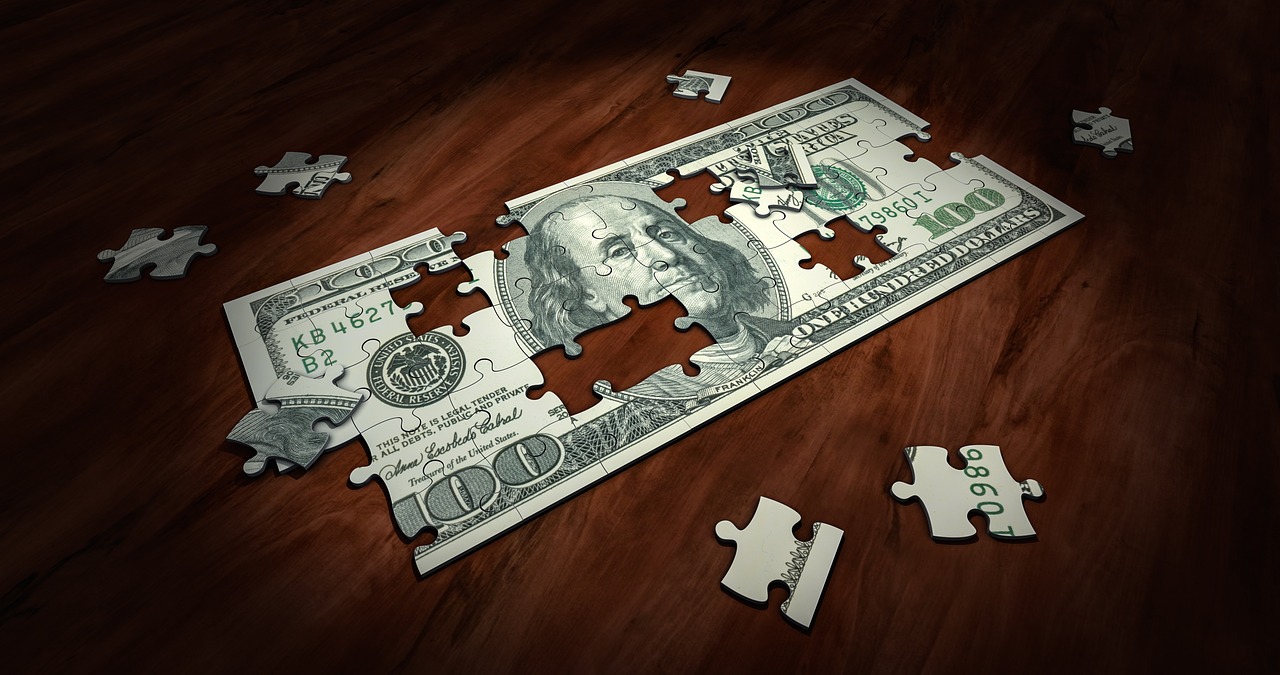
However, data issues always come to bite you in the long run. Just from the security and reliability points of view, you absolutely must be on top of your data happenings. Data lineage gives you that ability. The more granular data lineage is, the easier your life will be when things go wrong with your data.
Inevitably, you will have to implement data lineage, but then someone will have to code it from scratch. Data lineage must go all the way across the data from the source to the end point and cover the data at the lowest level regardless of the types. It should be the same granularity for all stakeholders, so everyone works off the base baseline. You will then have a much greater confidence in your data estate.
Implementing data lineage is not a simple job. You need to set and build in data quality and monitoring policies for all dataflows. Depending on your resources, this can be a daunting task. It is much trickier to implement if you are doing live data streaming. There are some tools available on the market that can help you with the task, but you need to make sure they can work well with the rest of your data estate and give you sufficient granularity.
Since we have encountered data lineage issues on more than one occasion, we made data lineage an integral part of our solution. We do DataOps, and data lineage is DataOps. At IOblend, we made sure that the most granular data lineage is available to you ‘out-of-the-box’. It starts at record level with the raw data and maps the transformations all the way to the end target. Our process utilises the power of Apache Spark™ but requires no coding whatsoever on the user’s part. Just visually design your dataflow and data lineage is applied automatically, every time.
Once applied, you can trace data lineage via IOblend or any other analytical tool you may use at your data end points. No hassle. Your data citizens will always have the full confidence in the quality of their data.
IOblend – make you data estate state-of-the-art
Stay safe and catch you soon
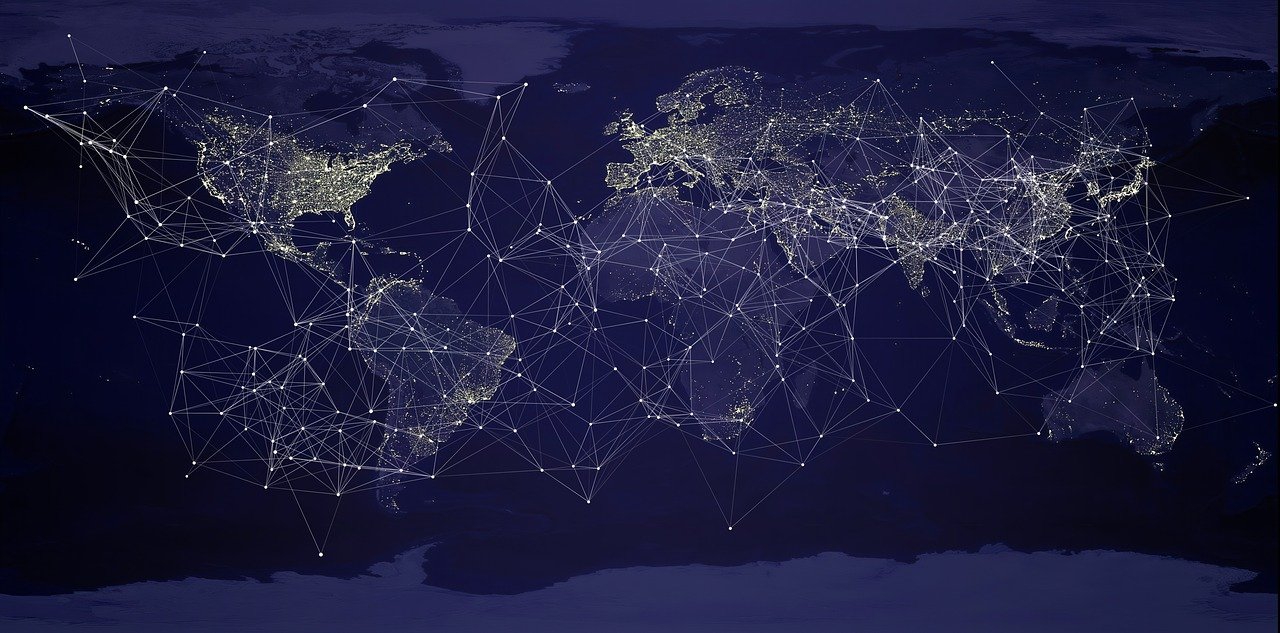
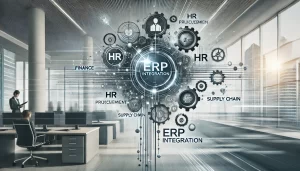
The ERP Shortcut: How to Integrate Faster Than You Think
IOblend was designed with one mission in mind: to simplify data integration. We deliver complex, real-time multi-system syncing with ERP in under a week.
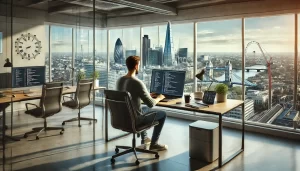
IOblend seamlessly powers real-time multi-system integration
The adoption of IOblend significantly improved our data transformation capabilities, allowing for efficient and secure data integration between multiple systems
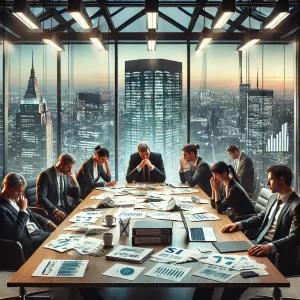
The AI Hype Trap: Why Overblown Promises Backfire
AI and GenAI adoption must make a visible and material positive impact on the business or it’s a waste of money.
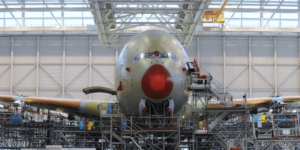
The Art of Assembly: Where Data Meets Conveyors
Manufacturing is all about getting the most out of automation, skilled workforce, and data. Data helps drive the decisions that keep everything running smoothly
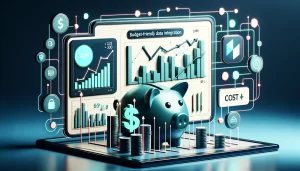
Saving Cents on Data Sense: Less Cost, More Value
No company is immune from the pains of data integration. It is one of the top IT cost items. Companies must get on top of their integration effort.
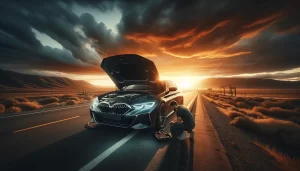
Operational Analytics: Real-Time Insights That Matter
Operational analytics involves processing and analysing operational data in “real-time” to gain insights that inform immediate and actionable decisions.