Keeping it Fresh: Don’t Let Your Data Go to Waste
Data hoarding has been a growing trend over the past decade with the advent of the Cloud and cheap storage. Companies collect as much data as they can – I mean why not if it’s not costing you a lot? Who knows when you may need it?
But when you do, it is not easy to process this data for analytical consumption. The businesses don’t even always know what data they are collecting exactly. Often, there is no clear owner for it and no simple way to identify what the data actually represents. So, it just “rots” in storage until some distant future date.
In this state, the data is useless. If the business is not making decisions from their data, it is a waste of space and money to keep collecting it. The goal is to put that data to good use. On a continuous basis. If there is value in data, you must put it to work. The currency of success is no longer in just having vast amounts of data but in using it effectively to drive decisions.
Best before date
We have done many a data discovery phase with clients. It’s rarely straightforward to uncover the data “riches” and to put proper management and governance policies around it. The data tends to sit in multiple systems, in various formats and slowly decaying. Most of that data is normally too far gone for anything other than a trip down the memory lane.
Usable data can go two-three years back, depending on the use case. It is still considered “fresh” to offer actionable insights, especially in strategic planning cases or trend analyses. In faster-paced applications like real-time decisioning and automation, the data can be out of date withing seconds. For instance, in phased array weather radar applications, a five-minute refresh produces inaccurate storm cloud mappings. The weather moves fast, and the data goes stale within seconds.
Decisions that have an impact today and in the future are based on fresh data. What your business did five-ten years ago might be interesting as a history lesson. An auditor will likely be keen to dig into your annals too. But stale data won’t help you determine your sales figures for the next month or drive your three-year expansion strategy. The data you need to make those decisions can only ever be fresh. It must be readily available, relevant, trustworthy, and current to be of any practical use. Otherwise, it loses its value.
Data freshness, fundamentally, means how current and accurate your data is. It’s not just a matter of having data. But having data that reflects the latest changes, trends, and information.
The freshness factor
It was great to see that what we preach about data freshness came to light so prominently at the recent Google Cloud AI Ignite event. The notion of data freshness has become critical with the advent of GenAI technologies. Once the models are trained, they need to be fed with new data to make sure they remain relevant.
In the world of data analytics and GenAI, freshness isn’t just a nice-to-have; it’s a critical success factor. Fresh data is the key for making informed decisions. It drives the understanding of market trends in real-time., It provides customers with what they need before they even know they need it. It’s about turning the vast ocean of “perishable” data into actionable insights that will drive a business forward.
Businesses often start with the best intentions, accumulating data to better understand their market and customers. However, without using fresh data, they can quickly find themselves making decisions based on outdated information. It’s akin to driving on a newly built road without the car satnav getting updated. You have no idea where you are heading.
In aviation, we often used planning and optimisation tools that consumed data from over a year in the past. It took so long to process and calibrate the tools with fresh data that this was the least bad option. But the world of aviation is notoriously fast-evolving, so the planning data can get stale after even a few weeks. Stale data severely diminished the impact of data-driven decisions. We had to rely on gut feel and expertise to compensate. You are always planning two to five years ahead using a year-old data (when it was fully updated and cleaned) at best.
Data misalignment
Not all data is equally fresh at the same time. Data generally resides in a central warehouse, departmental systems and disparate spreadsheets with potentially vastly different re-fresh cycles. How do you bring such data together in a coherent way for wider consumption?
You do want fresh-enough data that makes a material difference to your analysis (e.g. a competitor leaving or entering the market). But some sources may update half as often as the others. Does your dashboard still reflect the true picture when one of your sources has updated and the others have not? It’s not straightforward at all to decide on the appropriate logic in these cases.
The challenge lies around integrating the latest arriving data into the ongoing analytics process. Both from a logical perspective and as a technical challenge. It’s rare to see businesses serve data from multiple systems, data sources and departments in a unified and automated way. Sure, modern automated data processes involving sales and CRM do collect and serve fresh data. In many other business area, it’s coffee and long analyst hours to combining several data sources in a spreadsheet.
Whatever the refresh cycles are, all usable data should be made available with appropriate attributes, metadata and lineage in a coherent way. Automatically. Once the business agrees what the integration rules are, the rest should be a simple technical exercise.
Real-time freshness
Transitioning from stale data practices involves implementing robust data management processes and systems. Businesses must start leveraging Change Data Capture (CDC), adopting real-time analytics and implementing advanced data stitching techniques (e.g. chained aggregations). It’s a transformation that moves businesses from reacting to the past to anticipating the future.
Naturally, freshness depends on the use case. In some applications, fresh data can be a year old. In others, it must be sub-second latency, event driven. For instance, in the realm of e-commerce, inventory management systems rely on the most current data to reflect stock levels accurately. Imagine the customer dissatisfaction that could arise from ordering a product shown as available, only to find out it’s been out of stock for hours. Happened to me a few times!
Google sees stale data as one of the biggest obstacles to successful implementation of GenAI in enterprise applications. With fresh data, businesses can detect emerging trends, adapt to market changes swiftly, and personalise customer experiences on the fly.
The economic implications of leveraging fresh data are profound. For retailers, it means always having the right stock levels to meet customer demand. For financial institutions, it means making smarter investments and reducing risk. Across every sector, fresh data can be the difference between profit and loss, success and failure.
Practical steps to embrace data freshness
Organisations looking to improve their data freshness should invest in robust data management and integration tools that automate the process as much as possible. For example, solutions like our IOblend automate CDC and manage Slowly Changing Dimensions (SCD), enabling businesses to efficiently capture changes in their data sources automatically. On top of that, you can embed the transformation logic within the data pipeline itself, so that the updates happen in-transit and serve consumption-ready data to your systems.
This not only ensures data freshness but also reduces the workload on IT teams and dev cost. This approach is particularly valuable in environments where decisions must be made quickly and based on the latest information.
Invest in the right tools: Technologies like CDC and real-time analytics platforms are essential for maintaining data freshness.
Cultivate a data-driven culture: Encourage everyone in the business to understand the value of fresh data and make decisions based on the latest insights. Develop the process of updating the data that is out of synch with each other. Embed the culture of close collaboration across data owners, devs and consumers.
Automate data processes: Reduce manual handling to minimise errors and delays in data processing. Tools like IOblend are especially good at real-time data processing. And when combined with the cloud powerhouses like Snowflake, keeping the data fresh and readily available for analytics is easy.
Analytics requires relevant data to inform business decisions. In this case, relevant data means the data that is recent enough and of sufficient quality to be effective in decision-making. By employing the right mix of technologies, processes, and expertise, organisations can ensure that their data is a true reflection of the current state of affairs. This, in turn, will lead to improved operational efficiency, enhanced customer satisfaction, and ultimately, a competitive edge in the digital marketplace.
If you want to learn more about how IOblend helps to keep data fresh, please reach out to us. Tag me on LI if that’s easier. We are always happy to assist.
IOblend presents a ground-breaking approach to IoT and data integration, revolutionizing the way businesses handle their data. It’s an all-in-one data integration accelerator, boasting real-time, production-grade, managed Apache Spark™ data pipelines that can be set up in mere minutes. This facilitates a massive acceleration in data migration projects, whether from on-prem to cloud or between clouds, thanks to its low code/no code development and automated data management and governance.
IOblend also simplifies the integration of streaming and batch data through Kappa architecture, significantly boosting the efficiency of operational analytics and MLOps. Its system enables the robust and cost-effective delivery of both centralized and federated data architectures, with low latency and massively parallelized data processing, capable of handling over 10 million transactions per second. Additionally, IOblend integrates seamlessly with leading cloud services like Snowflake and Microsoft Azure, underscoring its versatility and broad applicability in various data environments.
At its core, IOblend is an end-to-end enterprise data integration solution built with DataOps capability. It stands out as a versatile ETL product for building and managing data estates with high-grade data flows. The platform powers operational analytics and AI initiatives, drastically reducing the costs and development efforts associated with data projects and data science ventures. It’s engineered to connect to any source, perform in-memory transformations of streaming and batch data, and direct the results to any destination with minimal effort.
IOblend’s use cases are diverse and impactful. It streams live data from factories to automated forecasting models and channels data from IoT sensors to real-time monitoring applications, enabling automated decision-making based on live inputs and historical statistics. Additionally, it handles the movement of production-grade streaming and batch data to and from cloud data warehouses and lakes, powers data exchanges, and feeds applications with data that adheres to complex business rules and governance policies.
The platform comprises two core components: the IOblend Designer and the IOblend Engine. The IOblend Designer is a desktop GUI used for designing, building, and testing data pipeline DAGs, producing metadata that describes the data pipelines. The IOblend Engine, the heart of the system, converts this metadata into Spark streaming jobs executed on any Spark cluster. Available in Developer and Enterprise suites, IOblend supports both local and remote engine operations, catering to a wide range of development and operational needs. It also facilitates collaborative development and pipeline versioning, making it a robust tool for modern data management and analytics
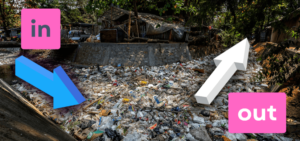
Data Quality: Garbage Checks In, Your Wallet Checks Out
Data quality refers to accuracy, completeness, validity, consistency, uniqueness, timeliness, and reliability of data.
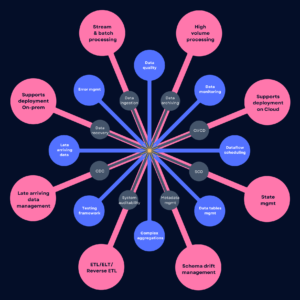
IOblend: State Management in Real-time Analytics
In real-time analytics, “state” refers to any information that an application remembers over time – i.e. intermediate data required to process data streams.
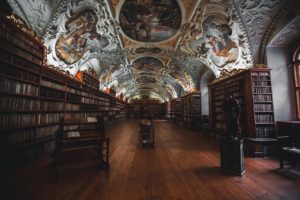
Data Lineage: A Data Governance Must Have
Data lineage is the backbone of reliable data systems. As businesses transition into data-driven entities, the significance of data lineage cannot be overlooked
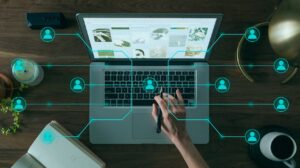
IOblend: Simplifying SCD for Real-Time Analytics
Businesses rely on accurate, up-to-date data to make informed decisions, which is why understanding and managing slowly changing dimensions (SCDs) is crucial.
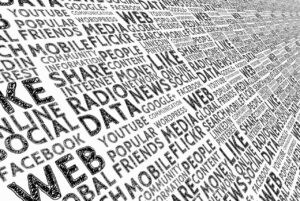
Metadata Management Made Simple with IOblend
MetadataIn today’s data-driven world, information reigns supreme. Businesses and organizations are constantly seeking ways to extract valuable insights from their data to make informed decisions. One often overlooked but essential aspect of this process is metadata. Metadata is the unsung hero that empowers data management, analytics, and decision-making.In this blog, we will delve into the
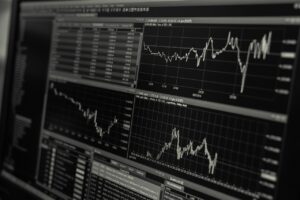
Change Data Capture: IOblend’s Seamless Approach
Change Data Capture In the fast-paced world of data management, staying ahead of the curve is not an option, it’s a necessity. Change Data Capture (CDC) is the secret weapon that allows businesses to keep pace with the constant flux of data. In this blog, we will delve into the world of CDC, explore different