Data Automation: investing pennies to save pounds
Today, we talk about the role Data Automation plays in data analytics and how it can save you a fortune. Let’s explore why this is the case.
When we talk about data analytics, we tend to focus mainly on exploratory analytics.
Explorative data analysis (EDA) enables data scientists and analysts to study underlying patterns, spot anomalies, identify important variables, and test hypotheses using statistical graphs, plots, and summary statistics. EDA is about making sense of data before making any assumptions or building predictive models. It’s fundamental in data science projects as it provides a roadmap for the analysis.
Steps in EDA
There is a caveat, however. EDA by nature is an experiment, driven by a business goal. It could be trying to better understand customer behaviour or model a logistical chain, etc. The business will have a hypothesis they try to prove or disprove with data. Alternatively, they might be looking to improve revenue or cut costs in a more informed manner.
The typical steps in EDA involve:
Understanding the data: Begin with a basic understanding of the data, including its source, size, and attributes.
Cleaning the data: Handle missing values, remove duplicates, and correct errors in the dataset.
Analysing distribution: Look at the distribution of individual variables using plots and summary statistics.
Exploring relationships: Examine how variables relate to each other and to the target variable, if applicable.
Performing advanced analyses: Depending on the complexity of the data, you may apply more sophisticated statistical tests or algorithms to uncover deeper insights.
So, EDA is mainly experimental modelling, snapshots of data from a variety of sources and a spreadsheet (typically).
EDA production challenges
The management gets excited when the analysts bring them good insights. They instantly want more of them and on a regular basis. But productionising the models into the BAU is not straightforward at all, unfortunately.
Transitioning EDA into a production-grade system is very different from a simple refresh of the data. The process requires addressing a number of challenges that do not typically affect EDA:
Scalability: Scalability issues arise from data storage, memory requirements, and the computational complexity of analysis techniques.
Reproducibility: Involves version control of data, code, and environment configurations to ensure that results can be replicated and validated.
Data drift detection: In a production environment, the system needs to be able to detect and adapt to data drifts to ensure that the insights remain accurate and relevant.
Performance monitoring: Production-grade analytics require continuous monitoring to ensure that the system performs as expected, like system failures, performance bottlenecks, and the accuracy of the analysis outputs.
Security and compliance: Ensuring data security and compliance with regulations (e.g., GDPR, HIPAA) is a major pain point, especially when dealing with sensitive or personal data. This includes implementing proper data access controls, encryption, and audit trails.
Integration with data pipelines: EDA models need to be integrated seamlessly with existing data pipelines and infrastructure.
Update frequency: EDA’s snapshot data may require low latency real-time streaming capabilities when in production.
Short-sightedness leads to false economy
In reality, many businesses lack vision, technical capabilities, money and patience to properly productionise EDA. Instead, they rely on data analysts and engineers to manually keep these models updated, which puts the company at risk on multiple fronts: data security breaches, manual errors, dependency on singular experts, scaling issues and high costs.
This is a big mistake companies make in hopes to save time and money. “Can you just refresh that report again? Like you did last time…” Doesn’t matter that it takes the poor sod three days to prepare the data. The manager doesn’t realise that such requests cost the company significant money. He/she has just wasted valuable SME time on a task that should have been done by a machine within minutes.
It’s always tempting to get results fast. I know it. I pushed for fast answers for years myself. And I produced plenty of “production” data reports derived from highly fragile EDA. It was never pretty. Data wrangling took 80% of the (expensive) time and we still had errors. The journey to automation was slow, however, as the business didn’t view the cost through the top-down lens. Automation sat with the IT budget, which was maxed out. Our time was paid for by Commercial and the cost was already sunk, so we stayed as we were. The business lost out overall and no one realised it.
Unfortunately, plenty of businesses are still in the same place with their analytics today.
Where does the money go?
Many companies think the answer to the ever-growing data demands is to bring in more analysts/engineers. It simply isn’t.
- You’re paying through the nose for what can be easily handled by a compact team of SMEs with proper tools at a fraction of the cost.
- Your data architecture becomes a spaghetti of complex pipelines, databases and siloed data products built using a multitude of tech and custom code.
- The valuable analytics resources spend most of their time on data wrangling tasks. Instead, they should be deployed on EDA and providing insights to the business. That’s what generates value, not the “behind-the-scenes” craftwork.
- Your bureaucracy grows because you have to manage ever larger teams of devs and analysts.
- Your company becomes less competitive because you are making decisions slower than your rivals do. And the cost of those decisions goes up.
- You can’t take advantage of the new opportunities fast enough to capitalise on them effectively.
These (often hidden) costs of bringing EDA to production are a material obstacle to generating business value.
Let’s remind ourselves what the purpose of data analytics is? It’s to enable the business to make better decisions that generate value. In simple words.
As such, data analytics should be viewed as a unit of “production”. No different from any other production inputs: raw materials, labour, capital. Data. The business succeeds by extracting value from using its resources in the most efficient manner. It’s important for businesses to carefully manage the costs associated with data analytics to ensure that the value produced exceeds the investment required. Companies sometimes forget that fact when pursuing potential gains.
Enter data automation
Just think about why it takes your data team so long to update your analytics in production. It’s never simply about going into a data source and sucking up all the data there into a lake/warehouse. The raw data can be incomplete, contain errors and duplicates, formats can be mixed up, etc. Lots of issues. The data teams try to figure out what those issues are, what caused them, whether they are they material, and so on. That can take hours or days. Now consider you have twenty different data sources that aggregate into a unified table.
This where data automation comes to play. It streamlines the workflow associated with data handling in production. It covers a whole suite of processes, including data ingestion, transformation, migration, management, governance and the generation of reports and insights. By leveraging tools designed for these purposes, companies can turn EDA into production systems with unprecedented speed and accuracy. Automation not only enhances productivity but also allows valuable employees to focus on more strategic tasks. For instance,
- In finance, data automation tools can process transactions, manage portfolios, and ensure compliance with regulations efficiently. Freed from the mundane, the SMEs can focus on further EDA to inform of new ways to make returns.
- In healthcare, patient records, treatment plans, and billing information can be managed seamlessly, improving patient care and operational efficiency. The doctors and nurses can now spend more time on actual patient care.
Automate as much as possible
- Data automation reduces manual data handling. Organisations will decrease labour costs associated with data ingestion, management and governance. Data is constantly generated in real-time. Some business functions rely heavily on low-latency data to operate. Automated processes are not only faster but also operate around the clock, increasing productivity without additional human resources (night and weekend shifts anyone?).
- Data automation reduces the risk of errors, which can be costly in terms of both financial repercussions and damage to an organisation’s reputation.
- Data automation also facilitates better resource allocation. By automating routine data tasks, employees can be redeployed to more strategic and value-added activities. This shift not only boosts employee satisfaction by eliminating monotonous tasks but also contributes to innovation and growth within the organisation.
- Additionally, data automation supports scalability. As the business grows, the volume of data it needs to manage increases. Automated systems can scale way more easily to accommodate this growth, without the increase in manual data processing costs.
A good example is real-time analytics and AI, where data automation is paramount. Real-time analytics are vital for applications that require immediate insights and actions, such as fraud detection, dynamic pricing, and real-time monitoring and alerting systems. Humans simply are unable to process and analyse such data manually and in real-time. How long can you stare at a live data dashboard before you lose your mind?
Data automation is simpler than you think
Data automation is a critical enabler of efficiency, accuracy, and strategic insight. It also considerably lowers your business cost when producing said insight. Many businesses are realising that they will have to automate but are not always sure how to approach it.
No worries. There is plenty of advice out there. Lots of consultancies and vendors specialise in data automation. We ourselves are experts in data automation at IOblend. Our entire business is built around helping companies make the most of their data through cost-effective and flexible automation. If you are interested to learn more about deploying data automation in your organisation, give us a shout. We are a friendly bunch and can help you get your data working for you quickly.
We are ISV partners with major data platforms and cloud providers and know our way around all kinds of data and systems. We can work with you directly or with your preferred delivery partners! Our interest is to set you on the path to data automation in the most efficient manner for your business.
IOblend presents a ground-breaking approach to IoT and data integration, revolutionizing the way businesses handle their data. It’s an all-in-one data integration accelerator, boasting real-time, production-grade, managed Apache Spark™ data pipelines that can be set up in mere minutes. This facilitates a massive acceleration in data migration projects, whether from on-prem to cloud or between clouds, thanks to its low code/no code development and automated data management and governance.
IOblend also simplifies the integration of streaming and batch data through Kappa architecture, significantly boosting the efficiency of operational analytics and MLOps. Its system enables the robust and cost-effective delivery of both centralized and federated data architectures, with low latency and massively parallelized data processing, capable of handling over 10 million transactions per second. Additionally, IOblend integrates seamlessly with leading cloud services like Snowflake and Microsoft Azure, underscoring its versatility and broad applicability in various data environments.
At its core, IOblend is an end-to-end enterprise data integration solution built with DataOps capability. It stands out as a versatile ETL product for building and managing data estates with high-grade data flows. The platform powers operational analytics and AI initiatives, drastically reducing the costs and development efforts associated with data projects and data science ventures. It’s engineered to connect to any source, perform in-memory transformations of streaming and batch data, and direct the results to any destination with minimal effort.
IOblend’s use cases are diverse and impactful. It streams live data from factories to automated forecasting models and channels data from IoT sensors to real-time monitoring applications, enabling automated decision-making based on live inputs and historical statistics. Additionally, it handles the movement of production-grade streaming and batch data to and from cloud data warehouses and lakes, powers data exchanges, and feeds applications with data that adheres to complex business rules and governance policies.
The platform comprises two core components: the IOblend Designer and the IOblend Engine. The IOblend Designer is a desktop GUI used for designing, building, and testing data pipeline DAGs, producing metadata that describes the data pipelines. The IOblend Engine, the heart of the system, converts this metadata into Spark streaming jobs executed on any Spark cluster. Available in Developer and Enterprise suites, IOblend supports both local and remote engine operations, catering to a wide range of development and operational needs. It also facilitates collaborative development and pipeline versioning, making it a robust tool for modern data management and analytics
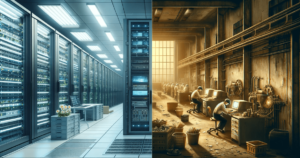
Data Automation: Investing Pennies to Save Pounds
Data automation is a critical enabler of efficiency, accuracy, and strategic insight. It also considerably lowers your business cost when producing said insight
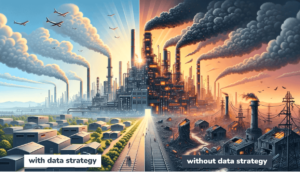
Data Strategy: Taking a Business View
Data strategy aligns data-related activities with the strategic goals of an organisation. It’s about turning data into value.
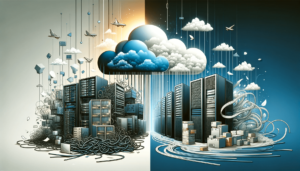
Out with the Old ETL: Navigating the Upgrade Maze
Today, we have tools and experience to make digital transformation easy. Yet, most organisations cling to their antiquated data systems and analytics. Why?
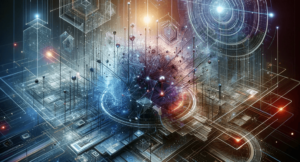
Smart Data Integration: More $ for Your D&A Budget
Data integration is the heart of data engineering. The process is inherently complex and consumes the most of your D&A budget.
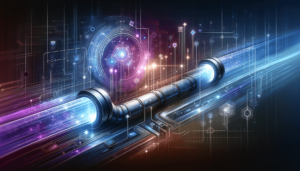
Data Pipelines: From Raw Data to Real Results
The primary purpose of data pipelines is to enable a smooth, automated flow of data. Data pipelines are at the core of informed decision-making.
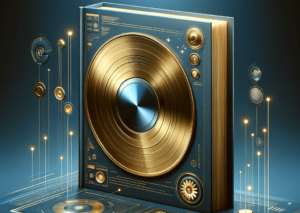
Golden Record: Finding the Single Truth Source
A golden record of data is a consolidated dataset that serves as a single source of truth for all business data about a customer, employee, or product.