Data Quality: When Garbage Checks In, Your Wallet Checks Out
We often hear these days that data is “the new oil”. We heard it mentioned more than a few times at the Big Data LDN debates. However, unlike oil, data’s value relies heavily upon its quality.
Data quality encompasses the accuracy, completeness, validity, consistency, uniqueness, timeliness, and reliability of data. High-quality data adheres to the correct format and remains free from errors, empowering organizations to make informed decisions with confidence instead of questioning the integrity of the underlying data.
Why is Data Quality important?
Informed Decision-Making: Good quality data underpins insightful analytics, aiding in informed decision-making. Inaccurate or incomplete data can lead to misguided decisions with potentially severe financial and operational repercussions.
Regulatory Compliance: Many sectors face strict regulatory requirements around data. Ensuring high data quality helps in adhering to these regulations and avoiding legal complications.
Customer Satisfaction: High data quality can significantly enhance customer satisfaction. Accurate information allows for better service, strengthening customer trust and retention.
Operational Efficiency: Data quality fosters efficiency by reducing errors that require correction, thereby saving time and resources. This is especially crucial is the industries highly reliant on automation, such as finance, aviation, manufacturing and healthcare.
Getting Data Quality wrong is costly
Poor data quality will heavily influence the outcomes of the decisions that are usually quite costly to remedy.
For instance, if your data is riddled with duplicates, your stats and trends will skew. This could result in bad (costly potentially) decisions.
If your sales data slowly changes over time and you don’t detect it, you will incur a hit on profitability.
In a real-time analytics example, data quality management is crucial as decisions are made instantly based on the incoming data. Bad quality data driving mission-critical actions can be catastrophic.
If you are integrating GenAI and LLMs into your organisation, bad quality data will wreak havoc on the generated output.
Data Quality in practice
In practice, managing data quality encompasses several key elements:
Data Governance: Setting clear policies and designating ownership regarding data quality are crucial steps. This involves specifying the individuals responsible for particular data sets and outlining the processes in place to guarantee accuracy and consistency. Presently, data contracts are emerging as a potential mechanism to integrate data quality right from the source.
Data Profiling: Assessing the data to understand its quality, including identifying inconsistencies and errors that need to be rectified.
Data Cleaning: Remedying data quality issues either manually or through automated processes, such as data de-duping or addressing “NULL” values.
Data Monitoring: Continuously monitoring data to ensure it maintains the desired level of quality. Issuing targeted alerts to the relevant parties.
Who manages Data Quality?
Various organisations adopt diverse approaches to managing data quality, tailored to their particular needs, size, and industry regulations. These strategies span from formulating data governance policies to utilising advanced tools and technologies for data quality management.
Rightly or wrongly, the responsibility for data quality often falls within the domain of Data Governance teams, comprising data stewards, data managers, and sometimes a Chief Data Officer (CDO). These teams ensure that data throughout the organization adheres to the set quality standards and compliance requirements.
We believe that embedding data quality within the overall data culture, rather than merely assigning it to a standalone team, is the way forward. While the designated team can offer overall governance and oversight, the onus of producing high-quality data should lie with every department.
Adopting this stance will significantly enhance data quality, diminish the necessity for its constant management, and expedite decision-making processes.
If good quality data is key to business success, why are we constantly debating it?
It seems that the pursuit of speedy delivery often overshadows data quality management. The priority often shifts towards generating rapid insights and swiftly moving data products into production, rather than investing effort in ensuring production-grade data quality beforehand. This aspect of management is perceived as bureaucratic, burdensome, and deemed unnecessary for many data projects.
What are the key drivers of the lack of wider adoption of data quality?
Desire to get results fast: It works fine now, right? Let’s run with it. Worry about the issues later.
Lack of self-discipline: Some organisations lack the rigour to get on top of their data issues. They would do nothing until it becomes impossible to ignore them. Kicking the can down the road.
Technical limitations: We have plenty of tech to do production grade data quality management. However, not everyone has the full suite of such functionality available to them or they lack the expertise to implement/develop in-house solutions. Interestingly, it is often seen quicker to do data quality manually at every re-fresh than invest in automation.
Cost: Implementing data quality policies and associated technologies can be expensive. The costs include software, hardware, and possibly consulting and ongoing maintenance fees.
Manpower: The implementation requires skilled personnel to manage and maintain the data quality processes. Finding and hiring the right talent can be challenging and expensive.
Lack of time: Implementing data quality measures can be a complex and time-consuming process, especially in large organisations with vast amounts of data or those with entrenched data management practices that may be outdated or inconsistent.
Resistance to Change: There might be resistance from employees who are accustomed to existing processes and systems, even if they are flawed or inefficient. Fear of the unknown or a lack of training and education about data quality management can contribute to this resistance.
Lack of Executive Buy-in: Sometimes, there’s a lack of support or understanding from the executive leadership regarding the importance of data quality, making it difficult to secure the necessary resources and prioritisation.
Fear of Uncovering Issues: Unveiling data quality issues can sometimes expose other organisational problems or past mistakes, which companies might prefer to keep under wraps.
In practice, most companies will have a combination of these challenges affecting their journeys to better data quality. The latter three are the worst of them all. If there is no real desire to change for the better, no matter what technology or however many resources you deploy, the effort will fail. These organisations only tend to react after they get stung by expensive blunders.
How to succeed in implementing Data Quality Management (DQM)?
When companies recognize the significance of maintaining high-quality data, they are faced with the decision of choosing an approach for implementing Data Quality Management (DQM) across their data landscapes.
Regardless of the maturity of the enterprise’s data landscape, the complexity of its data and systems, or the level of expertise in executing and managing data quality, the following steps will prove to be helpful:
First, secure the executive buy-in. If the top management is onboard, you can drive the DQ culture change through all layers of the organisation.
Establish a Data Governance framework: Develop a robust data governance framework that defines roles, responsibilities, and processes for managing data quality within the organisation.
Identify and Define Key Metrics: Define key metrics and standards for data quality, including accuracy, consistency, completeness, reliability, and timeliness.
Perform Data Quality audits: Conduct regular data quality audits to assess the current state of data quality and identify areas for improvement.
Implement Data Quality Management tools: Utilise data quality management tools and software capable of automating numerous facets of data quality upkeep, including data validation, monitoring, and cleaning. This element is particularly crucial if you engage in real-time analytics or operate production systems where decisions are automated, and errors can cause significant adverse effects.
Continuous monitoring and reporting: Establish continuous monitoring and reporting mechanisms to ensure data quality remains high and to quickly identify and rectify any emerging issues.
Data Quality Education and Training: Provide training and resources to staff on the importance of data quality and best practices for maintaining it. Can’t stress this more.
Implement Data Stewardship: Appoint data stewards responsible for overseeing data quality within different parts of the organisation.
Implement Data Contracts: Create validation rules to ensure that data is formatted correctly and is accurate and reliable before it enters your estate – at the source ideally, as you will have issues propagating down your pipelines.
Leverage Machine Learning and AI: Utilise artificial intelligence (AI) and machine learning (ML) for ongoing data quality improvement, anomaly detection, and automated data cleaning.
Maintain Documentation: Document data definitions, processes, and quality standards to maintain consistency and to provide a reference for staff.
Feedback Loops: Establish feedback loops with end-users and data providers to continually improve data quality processes based on user feedback and experiences.
Periodic Review and Update: Regularly review and update data quality strategies to keep them aligned with organisational objectives and evolving data needs.
Through meticulous planning and execution of a comprehensive data quality strategy, organisations can markedly improve the accuracy, reliability, and usefulness of their data, thereby promoting enhanced decision-making and operational efficiency.
Moreover, this doesn’t have to commence as an overwhelming Big Bang project. Initiating on a smaller scale and then expanding across the organization with each success can be a more manageable approach. This process is iterative — with time and better management practices, Data Quality will progressively improve.
IOblend simplifies Data Quality Management
At IOblend, the essence of data quality management wasn’t just a thought, it was a driving force behind crafting our solution. Once the nods of approval are in and the governance policies are established, bringing data quality into action should never feel like a technical marathon.
We see data quality as a staple in every data pipeline, which is why we’ve knitted data quality management features right into IOblend, throwing a strong arm around our data teams. Our eyes were fixed on automation and making the implementation a breeze, all while handing over the reins to users to enforce their own data quality policies and methods.
What makes IOblend stand a cut above in the landscape is its snug-fit approach to data quality management. By nestling data quality checks within the data integration pipelines, we make sure data quality isn’t an afterthought but an integral part of every element of the dataflow. Our game isn’t just about Change Data Capture (CDC), lineage, schema management, or metadata – we do it all!
We fully understand how the dollar signs and the engineering effort needed to implement and manage data quality can send shivers down the spine of many companies. The market is brimming with tools and platforms catering to data quality. But our two cents? Keep the tool count to a minimum. A swelling toolkit only cranks up the complexity and cost for you.
Aim for a Swiss Army knife of a tool that juggles multiple functions instead of a one-trick pony. If your ETL (Extract, Transform, Load) process can handle data quality management, you’ll find yourself sweating less over integration, security, and management.
Don’t just get swayed by the flashy headline numbers – drill down to the total cost of ownership. And remember, there’s no such creature as a “free” tool. They all require engineering effort behind the scenes, costing you in time, effort and opportunity.
We made it easy to try IOblend’s data quality capabilities by offering a FREE Developer Edition. Download and see for yourself how quickly you can build production-grade data pipelines and flow quality data into your analytics.
Managing data quality in the context of complex data landscapes like those encountered in cloud data migration projects is a multifaceted challenge, effectively addressed by IOblend. The crux of managing data quality lies in its dimensions: accuracy, completeness, validity, consistency, uniqueness, timeliness, and reliability. In practice, this involves establishing robust data governance frameworks, engaging in data profiling and cleaning, and continuously monitoring data quality. IOblend simplifies this process by integrating data quality management into every data pipeline, ensuring that it is an integral part of the dataflow rather than an afterthought. This approach includes automated features for data validation, monitoring, cleaning, and even leveraging AI and ML for ongoing improvement. By reducing the complexity and cost of data quality management and embedding these processes within the data integration pipelines, IOblend offers a streamlined and efficient solution to managing data quality, crucial for making informed decisions and ensuring regulatory compliance.
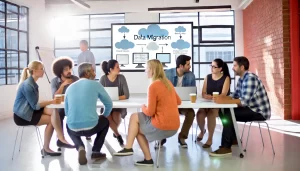
Why IOblend is Your Fast-Track to the Cloud
From Grounded to Clouded: Why IOblend is Your Fast-Track to the Cloud Today, we talk about data migration. Data migration these days mainly means moving to the cloud. Basically, if a business wants to drastically improve their data capabilities, they have to be on the cloud. Data migration is the mechanism that gets you there.
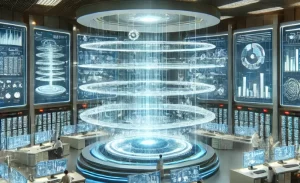
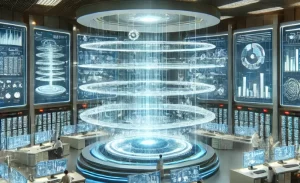
Data Integration Challenge: Can We Tame the Chaos?
The Triple Threats to Data Integration: High Costs, Long Timelines and Quality Pitfalls-can we tame the chaos? Businesses today work with a ton of data. As such, getting the sense of that data is more important than ever. Which then means, integrating it into a cohesive shape is a must. Data integration acts as a
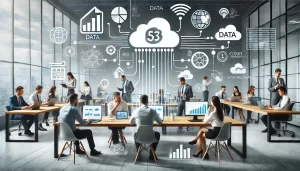
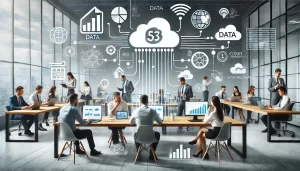
Tangled in the Data Web
Tangled in the Data Web Data is now one of the most valuable assets for companies across all industries, right up there with their biggest asset – people. Whether you’re in retail, healthcare, or financial services, the ability to analyse data effectively gives a competitive edge. You’d think making the most of data would have
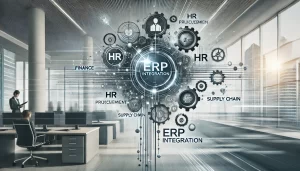
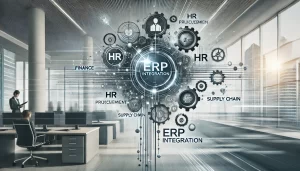
The ERP Shortcut: How to Integrate Faster Than You Think
IOblend was designed with one mission in mind: to simplify data integration. We deliver complex, real-time multi-system syncing with ERP in under a week.
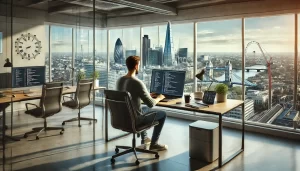
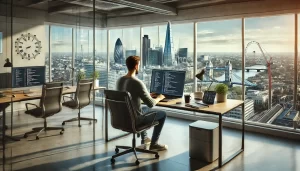
IOblend seamlessly powers real-time multi-system integration
The adoption of IOblend significantly improved our data transformation capabilities, allowing for efficient and secure data integration between multiple systems
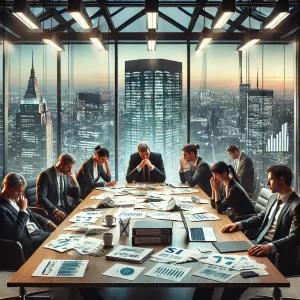
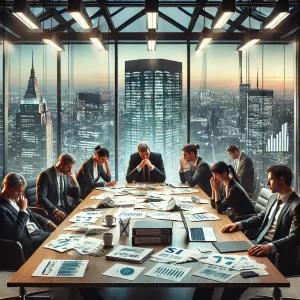
The AI Hype Trap: Why Overblown Promises Backfire
AI and GenAI adoption must make a visible and material positive impact on the business or it’s a waste of money.