Dynamic decisioning
We continue exploring the topics of operational analytics (OA) in the aviation industry.
Data plays a crucial role in flight performance analytics, operational decisioning and risk management. Real-time data enhances them.
The aviation industry uses real-time data for a multitude of operational analytics cases: monitor operational systems, measure wear and tear of equipment, power dynamic product pricing/packaging, feed AI/ML algorithms, or measure flow of resources and people through airports. Real-time analytics allow them to process data on the fly and act upon it pro-actively, like address equipment deterioration before it breaks down or alter the seat pricing dynamically depending on customer demand. This is called dynamic decisioning.
The benefits of dynamic decisioning in operational settings are considerable. Just think about how much money is saved by forecasting accurately and preventing component failure by swapping it out just before it fails. Millions in the case of engine failures. This way you get the highest use from the component without any unscheduled downtime (AOG events). Another example is monitoring live flight data feeds at critical flight stages (e.g. approach/landing) to alert the aircraft of any developments that may affect a safe operation.
Point solutions run aviation
But these examples are not a common practice yet. Some operators are further along the real-time analytics journey, but mainly in certain specific areas – point solutions. Like the aircraft engine suppliers offering real-time analytical systems to monitor performance and component deterioration. They monitor engine performance and forecast useful life by comparing it to the historical data set of the same model engines used by other operators under the similar usage conditions (if the data sample is big enough).
Or let’s consider dynamic seat pricing systems that match demand to capacity. These would use historical sales data and a lot of static commercial reference data to match the price to the demand using proprietary algorithms.
Both are standalone solutions that rely on very careful and lengthy data integration, SME monitoring and manual interventions due to the system rigidities. When the seat pricing occurs, the only operational variables that will be fed are the seat capacity and range (i.e. X aircraft can operate Y route safely). No operational performance data beyond the estimated average cost of flight ever gets incorporated.
Today, the Operations planning use engine performance data to manually allocate aircraft to the routes. The idea is to match the most efficient aircraft to the mission, such as flying far with a heavy passenger load is better on more efficient engines. But the allocation happens late and plays no role in the pricing strategy. Flight Ops simply do not have the visibility of the exact fleet availability until very late in the planning phase. Sometimes not even up to the time of departure.
Unified perspective
But what if the aircraft performance data were used as a feed for the revenue management? Depending on the route type and sales progress, the exact aircraft allocation would allow the algorithm to adjust the sales price to generate the highest contribution. Simplified case, I know, but it demonstrates the options that become available to the business.
If you are fully aware of the entire fleet condition in real time, you could make optimisation decisions dynamically for the entire network all the way to the day of departure. Fleet planning, fleet allocation, scheduling and flight ops are all aware of what is happening to the aircraft at any given point. Network optimisation can happen in real time.
Industry data engineering challenge
Attaining these advanced capabilities is not simple, however. Companies need to step well outside of the BAU. They need to be able to build dynamic, unifying data capabilities that would work across the federated point solutions space and provide real-time insights. To achieve that, the data needs to be production-grade and interoperable from the get-go. Since the data flows in real time, you must be able to trust it is robust and reliable throughout the dataflow, source to destination. Decisions are made live – there is no questioning of the quality of the data.
This means multiple quality-ensuring processes must be applied to the streaming data also in real time, such as data logging at record level, error checking, drift monitoring, data lineage and management of late arriving data. On top of that, you need to be able to perform complex transformations and data enrichment from other systems, while ensuring the output of these data pipelines is robust too.
Today we have powerful frameworks like Apache Spark that allow creation and management of efficient, production-grade dataflows in real time. But there is a catch. To properly utilise such frameworks requires you to have a deep knowledge of them, supplemented by expert, specialised engineering skills for implementation. Such skills are hard to come by and cost a fortune.
That is what’s stopping aviation companies from making the transition to advanced analytics today.
IOblend makes the transition to OA much easier
What they usually end up with (depending on how big their budgets are) is a patchwork of point solutions. They solve a narrow scope of issues they were designed for, but take a considerable effort to set up, learn and manage. Upgrading or replacing them generally proves too difficult due to the way they were originally designed and how deeply integrated they become over time. Airlines would not touch their decades old revenue management systems even as they know they are “leaving money on the table”. Any “global” analysis occurs on subsets of data and is static. Decisions are made manually and take forever because it is so hard to figure out what’s happening where at any given point in time.
Still, the aviation industry will adopt advanced analytics. They will have no option but to do it to stay competitive and improve margins. Costs pressures never stop, and they can push the revenue streams to compensate only so far. Efficiency is paramount in this industry.
At IOblend, we are trying to make it easier and cost effective to make the transition. We speak “aviation” and “data” fluently. Get in touch so we can demonstrate how you can get started on the journey, even on a tiny scale. All aviation companies are sitting on brilliant ideas to improve their operations but lack resources or time to put them into action. We can help you bring them to life.
Improving airline operations and analytics can be significantly enhanced by leveraging real-time data and operational analytics (OA), as discussed in IOblend’s insights. The aviation industry, by adopting advanced data-driven approaches such as dynamic decisioning and real-time analytics, can optimize various aspects like flight performance, equipment maintenance, and dynamic pricing strategies. Real-time data processing allows for proactive adjustments, such as addressing equipment issues before failures occur or altering pricing based on customer demand. However, transitioning to such advanced analytics requires overcoming challenges in data engineering and integration. Frameworks like Apache Spark facilitate the creation and management of efficient, real-time data flows, yet they demand specialized skills. IOblend aims to simplify this transition by providing expertise in aviation and data, helping aviation companies utilize their data more effectively and make informed decisions to stay competitive and improve margins.
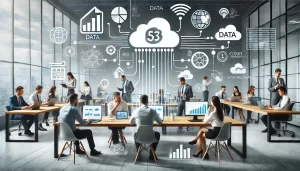
Tangled in the Data Web
Tangled in the Data Web Data is now one of the most valuable assets for companies across all industries, right up there with their biggest asset – people. Whether you’re in retail, healthcare, or financial services, the ability to analyse data effectively gives a competitive edge. You’d think making the most of data would have
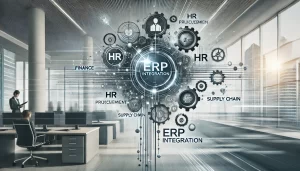
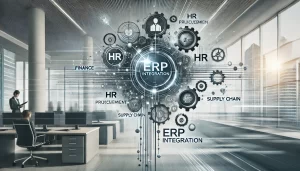
The ERP Shortcut: How to Integrate Faster Than You Think
IOblend was designed with one mission in mind: to simplify data integration. We deliver complex, real-time multi-system syncing with ERP in under a week.
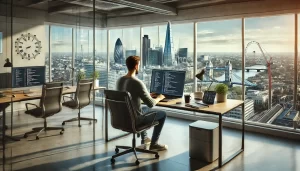
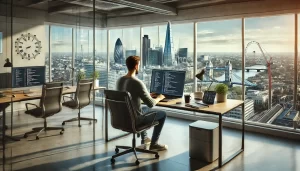
IOblend seamlessly powers real-time multi-system integration
The adoption of IOblend significantly improved our data transformation capabilities, allowing for efficient and secure data integration between multiple systems
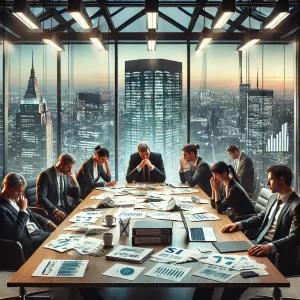
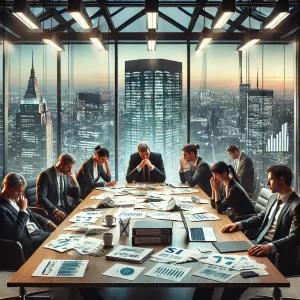
The AI Hype Trap: Why Overblown Promises Backfire
AI and GenAI adoption must make a visible and material positive impact on the business or it’s a waste of money.
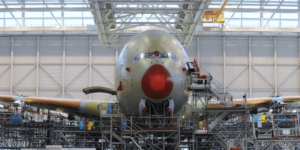
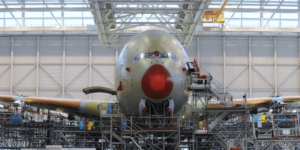
The Art of Assembly: Where Data Meets Conveyors
Manufacturing is all about getting the most out of automation, skilled workforce, and data. Data helps drive the decisions that keep everything running smoothly
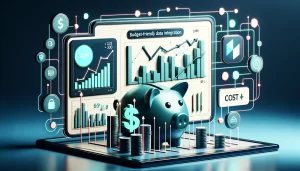
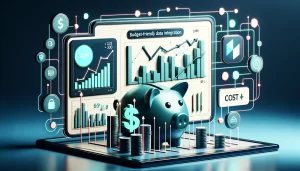
Saving Cents on Data Sense: Less Cost, More Value
No company is immune from the pains of data integration. It is one of the top IT cost items. Companies must get on top of their integration effort.