Mind the Gap: Bridging Data Shift Left: Unleashing Data Power with In-Memory Processing
💻 Did you know? Organisations that implement shift-left strategies can experience up to a 30% reduction in compute costs by cleaning data at the source.
The Essence of Shifting Left
Shifting data compute and governance “left” essentially means moving these processes closer to the data source, earlier in the data lifecycle. Instead of centralising everything in a data warehouse after data has been moved and transformed, more processing and control happen at the point of ingestion or even within transactional systems.
The Challenges of Traditional Data Management
Many organisations grapple with latency and complexity in their data pipelines. Extracting, transforming, and loading (ETL) vast datasets into a central data warehouse can be time-consuming and resource-intensive. This delay hinders real-time insights and agile decision-making. Furthermore, governing data that has passed through multiple stages and systems can become a tangled web, making it difficult to ensure quality, compliance, and security. Imagine a retailer trying to react to rapidly changing customer behaviour based on yesterday’s sales figures – they’re already behind the curve.
The Shift-Left Approach
The shift-left approach advocates for processing and governing data near its source. This means cleaning, transforming, and applying governance rules as early as possible in the data lifecycle. This allows for:
Reduced Latency: In-memory processing significantly reduces the time it takes to access and process data, enabling real-time analytics and decision-making.
Improved Data Quality: Cleaning and validating data at the source minimizes errors and ensures higher data quality.
Cost Savings: Processing data in-memory reduces the need for expensive data movement and storage.
Enhanced Governance: Applying governance policies early in the process ensures consistent and compliant data across the organisation.
Increased Agility: Faster data processing and improved data quality enable businesses to respond more quickly to changing market conditions.
IOblend: Empowering Shift-Left Data Processing
IOblend is a comprehensive DataOps solution designed to enable businesses to adopt a shift-left strategy in data processing. By facilitating early-stage data integration, transformation, and validation, IOblend ensures that data issues are addressed promptly, reducing downstream complexities and costs.
Key Capabilities:
In-Memory Compute: Leveraging a custom engine built on Apache Spark™, IOblend executes ETL pipelines in-memory, allowing for real-time data transformations without relying on expensive data warehouses. This approach supports processing data as it moves, enhancing efficiency and reducing latency.
Real-Time Data Integration: IOblend seamlessly integrates both real-time streaming and batch data from diverse sources, including JDBC, APIs, ESBs, dataframes, and flat files. Its architecture supports Change Data Capture (CDC), ensuring that the most recent data is always available for analysis.
Automated Data Quality Management: Built-in data quality features, such as schema validation, deduplication, and error handling, ensure the reliability and validity of data throughout the pipeline. This automation reduces manual intervention and accelerates data readiness.
Low-Code/No-Code Pipeline Development: Full data modelling capabilities like the warehouse, but without a need for one. Users can apply business logic using SQL or Python, streamlining the development process and enabling rapid deployment. No limitations on functionality.
Flexible Deployment Options: Whether on-premises, in the cloud, or hybrid environments, IOblend’s decoupled storage and compute architecture allows for adaptable deployment strategies, ensuring optimal performance and cost-effectiveness. Run computes on the most cost-effective infra (e.g. on-prem data centres, EC2, etc) and save a fortune on data processing,
By shifting data processing to earlier stages, IOblend empowers organizations to detect and resolve data issues promptly, streamline operations, and accelerate time-to-insight.
Ready to shift left and unlock the power of your data? Contact us today!
IOblend: See more. Do more. Deliver better.
IOblend presents a ground-breaking approach to IoT and data integration, revolutionizing the way businesses handle their data. It’s an all-in-one data integration accelerator, boasting real-time, production-grade, managed Apache Spark™ data pipelines that can be set up in mere minutes. This facilitates a massive acceleration in data migration projects, whether from on-prem to cloud or between clouds, thanks to its low code/no code development and automated data management and governance.
IOblend also simplifies the integration of streaming and batch data through Kappa architecture, significantly boosting the efficiency of operational analytics and MLOps. Its system enables the robust and cost-effective delivery of both centralized and federated data architectures, with low latency and massively parallelized data processing, capable of handling over 10 million transactions per second. Additionally, IOblend integrates seamlessly with leading cloud services like Snowflake and Microsoft Azure, underscoring its versatility and broad applicability in various data environments.
At its core, IOblend is an end-to-end enterprise data integration solution built with DataOps capability. It stands out as a versatile ETL product for building and managing data estates with high-grade data flows. The platform powers operational analytics and AI initiatives, drastically reducing the costs and development efforts associated with data projects and data science ventures. It’s engineered to connect to any source, perform in-memory transformations of streaming and batch data, and direct the results to any destination with minimal effort.
IOblend’s use cases are diverse and impactful. It streams live data from factories to automated forecasting models and channels data from IoT sensors to real-time monitoring applications, enabling automated decision-making based on live inputs and historical statistics. Additionally, it handles the movement of production-grade streaming and batch data to and from cloud data warehouses and lakes, powers data exchanges, and feeds applications with data that adheres to complex business rules and governance policies.
The platform comprises two core components: the IOblend Designer and the IOblend Engine. The IOblend Designer is a desktop GUI used for designing, building, and testing data pipeline DAGs, producing metadata that describes the data pipelines. The IOblend Engine, the heart of the system, converts this metadata into Spark streaming jobs executed on any Spark cluster. Available in Developer and Enterprise suites, IOblend supports both local and remote engine operations, catering to a wide range of development and operational needs. It also facilitates collaborative development and pipeline versioning, making it a robust tool for modern data management and analytics
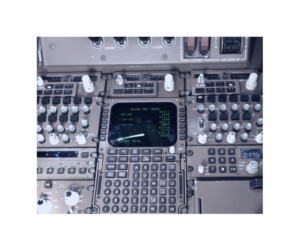
Unlock new capabilities with real time ACARS data
In this short article we are looking at one of the key data sources for the aviation industry – ACARS – and how IOblend helps to unlock new analytical capabilities from it.
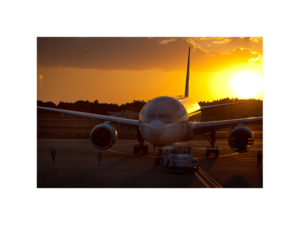
Time to automate your airline’s DOC data
How to automate Direct Operating Cost (DOC) data collection, processing and serving with IOblend.
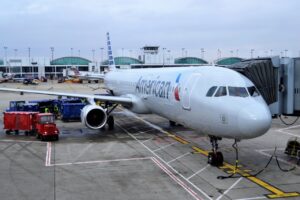
Automate airline fuel data collection & management
Collecting and managing airline fuel data is complex and time consuming. IOblend can greatly streamline the process and enable real-time decisioning.
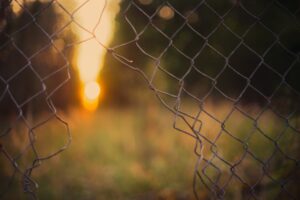
The Data Mesh Gotchas!
I think most practitioners in the data world would agree that the core data mesh principles of decentralisation to improve data enablement are sound. Originally penned by Zhamak Dehghani, Data Mesh architecture is attracting a lot of attention, and rightly so. However, there is a growing concern in the data industry regarding how the data
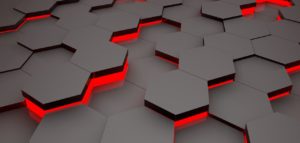
IOblend Data Mesh
IOblend Data Mesh – power to the data people! Analyst engineering made simple Hello folks, IOblend here. Hope you are all keeping well. Companies are increasingly leaning towards self-service data authoring. Why, you ask? It is because the prevailing monolithic data architecture (no matter how advanced) does not condone an easy way to manage the
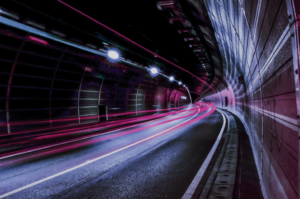
Data lineage is a “must have”, not “nice to have”
Hello folks, IOblend here. Hope you are all keeping well. There is one thing that has been bugging us recently, which led to the writing of this blog. While working on several data projects with some of our clients, we observed instances when data lineage had not been implemented as part of the solutions. In