Bridging Worlds: When Data Science Meets Domain Expertise
Companies are obsessed with being “data-driven”. They increasingly say they rely on data to drive decisions. They widely boast that data increases their sales and lowers the costs. They say data is at the centre of their business decision-making. All strive to use more data as part of their daily activities.
In the data industry we love coming up with new catchy terms. For data-driven, I’ve seen at least three flavours: data-informed, data-enabled and data-led. Whatever. The key here is that companies are bringing data to the forefront of their decision-making. This is a very good thing. Data-driven companies tend to outperform their competitors who are lagging behind.
The use of data for decisions is nothing new, however. Despite what the hype may lead us to believe. Businesses have been at it for decades. “Databases” existed on paper if you really want to go back. Of course, we have more quantity and variety of data available to us today. More data sources that generate it. Mostly in digital form too. We also now have much better tools to access and process it.
But the practice of using data for decision-making has been around for a long time. It’s just the use of it is not uniform across organisations and depends highly on the domain and culture.
The corporate sea
Most business insights are produced within domains, using highly localised (read siloed) tools and systems. The knowledge resides with the domain experts. They are career professionals who specialise in a particular area. Finance people do finance. They do it better than anyone else (often must obtain formal qualifications). HR specialists do recruitment, training, onboarding, payroll. Sales and marketing drive revenue.
Each area focuses on their remit, producing or consuming data that relates to their core activities. They all have different data requirements, use different systems, report to different managers, adhere to different KPIs and cater to different audiences. Islands in the corporate sea.
Underpinning this “sea” are central data teams that support all departments with their data and technology needs. These folks are data professionals. They know all things related to infrastructure, data engineering, management and governance. They architect efficient data estates, run data warehouses, do data migrations, perform complex integrations, etc. They manage data at an enterprise level.
Domains vs central data teams
Domain experts are very good at making decisions relating to their domains. They are well-versed in the data they use. They understand its limitations and know what “good” looks like in terms of data quality. But the domains know nothing about data technology.
On the other hand, the central data teams know everything about data technology. But they possess very little domain knowledge. They view all data the same, regardless of what it is used for downstream. It flows from source to consumer, must be managed, governed, and adheres to the domain requirements. What the domains do with it is generally not their concern. In this regard, central data teams act a support function for the domains, interacting only when things go wrong (i.e. service desk) or new data/system requirements arise (project delivery).
Yet, in the modern enterprise, we need to bring both the domain expertise and the data knowledge together. To get the most value from the data, the domains must be able to quickly obtain and iterate new data sources, seamlessly share data with other departments, develop apps and data products, etc. Without having the required technical expertise, they will never get there.
Understanding the divide
In most companies there exists a fundamental divide in perspectives and languages between business domain experts and data teams. Business domain experts are doing business things. They operate within a realm of strategic objectives and KPIs. Data teams, on the other hand, deal with data models, algorithms, and analytics, focusing on the “how” of data manipulation and interpretation.
The goal is to bring them together in a harmonious fashion to foster better collaboration and communication. So that everyone works towards the common set of objectives of adding higher business value.
Bridging the divide
How do we go about effectively bringing the data expertise and domain knowledge together? That’s a million-dollar question! Bridging the gap between these two realms isn’t straightforward at all (be very suspicious of claims that state otherwise).
But one thing is clear: businesses must bring these teams together. With the increasing demands for broader analytics use cases and the advent of GenAI, leaving things the way they were is unworkable in the long run.
There are several approaches to combining these skills, each with its own benefits and challenges. None are perfect and all require a strategic vision from the business top leadership.
Embedding data experts into domain teams
This approach, I find, worked best where I saw it implemented. But it requires time and careful implementation.
Pros:
- Data experts can learn the domain specifics directly from the source, ensuring that the technology solutions they develop are closely tailored to the actual needs of the domain.
- This approach fosters a collaborative environment where continuous feedback from domain experts can guide the development process in real-time.
- It encourages the data team to become more customer-focused, understanding the problems from the domain team’s perspective.
- Data experts will acquire domain that can help them in career progression.
Cons:
- There is a steep learning curve for the data experts to acquire domain knowledge, which might slow initial progress. If the business is in a hurry to deliver a solution, it will be difficult to do it this way in short-term.
- Data experts might not have the same level of passion or interest in the domain, which could affect their motivation and effectiveness.
Training domain experts in data and tech skills
There are certainly many technically apt domain experts out there. However, from what I’ve observed, the skills required to be a proper techie take years to achieve. A data engineer skillset takes a lot of effort to earn.
Pros:
- Domain experts are already deeply knowledgeable and passionate about their field, ensuring that any technological solutions developed are highly relevant and beneficial.
- This approach can be more efficient in terms of applying domain knowledge to solve problems with technology.
- It empowers domain experts to innovate within their field, potentially leading to groundbreaking advancements.
Cons:
- As mentioned, the learning curve for domain experts to acquire tech skills is significant.
- It might be challenging to find domain experts willing or able to develop the necessary tech skills.
- It takes years to acquire data engineering expertise.
Hybrid teams with cross-functional roles
On paper, this sounds like a sound approach. In practice, it’s very hard to implement effectively.
Pros:
- Combines the strengths of both approaches, leveraging deep domain knowledge and technical expertise.
- Encourages a culture of collaboration and mutual learning, enriching both the domain and tech teams.
- Facilitates a balanced perspective on problem-solving, ensuring both domain relevance and technological feasibility.
Cons:
- Requires more effort to manage effectively, as it involves coordinating a diverse group of professionals with different backgrounds and expertise.
- The success of this approach heavily depends on the willingness of individuals to collaborate and learn from each other.
- Roles change, people move jobs. Knowledge transfer is limited due to a lack of embedding.
- Strategic re-prioritisation may take the tech resources away from domains, losing all progress in the process.
The technology angle
But what if give the domain teams easy-to-use tools, train them and let them get on with their work themselves?
Pros:
- Domain experts can directly manipulate data, run analyses, and generate reports without waiting for technical support. This can significantly speed up the decision-making process.
- Self-service tools can be customised to fit the specific needs and preferences of the domain, making them more relevant and easier to use for domain experts.
- These tools can facilitate greater collaboration among domain experts, as they share insights, data visualisations, and models more easily.
- By engaging directly with data and analysis tools, domain experts can develop a stronger understanding of data science principles and techniques.
Cons:
- Ensuring that self-service tools are user-friendly while still powerful enough to handle complex analyses can be difficult. There’s a risk that the tools could be either too simplistic for meaningful insights or too complicated for domain experts to use effectively.
- With more individuals accessing and manipulating data, maintaining data quality and adhering to governance policies become more challenging. There’s a risk of data silos, inconsistencies, and breaches of data privacy regulations.
- Without a deep understanding of data science principles, there’s a risk that domain experts might misinterpret data or draw incorrect conclusions from their analyses.
- Developing, customizing, and maintaining these self-service tools can require significant investment in terms of time, money, and resources.
What’s the answer?
The best way, I believe, is to embed data experts into domains and empower them with appropriate tech. Over time, they will gain a sufficient understanding of the domain knowledge to help move the analytical dial.
The data experts will gradually disseminate their knowledge with the domains. They will manage the data, apply governance policies liaise with the central data teams. The domains, on the other hand, will be free to get on with what they do best. But this time, they will be fully supported and technologically empowered to iterate faster, make more informed decisions and drive the business forward. The domain and data experts working in harmony towards the common goals.
Bridging the gap between business domain expertise and data teams is not a one-off effort, mind. The senior management needs to understand this clearly. It is a continuous journey towards a more integrated, data-driven organisation. It requires strategic vision and investment in time and people. Only then, the business will truly become data driven.
If you are undertaking a journey to your data enlightenment, don’t hesitate to get in touch. At IOblend, we have many years of experience developing effective data capabilities in various organisations. We have developed cost-effective technological means to make the transitions to data-driven businesses easier.
IOblend presents a ground-breaking approach to IoT and data integration, revolutionizing the way businesses handle their data. It’s an all-in-one data integration accelerator, boasting real-time, production-grade, managed Apache Spark™ data pipelines that can be set up in mere minutes. This facilitates a massive acceleration in data migration projects, whether from on-prem to cloud or between clouds, thanks to its low code/no code development and automated data management and governance.
IOblend also simplifies the integration of streaming and batch data through Kappa architecture, significantly boosting the efficiency of operational analytics and MLOps. Its system enables the robust and cost-effective delivery of both centralized and federated data architectures, with low latency and massively parallelized data processing, capable of handling over 10 million transactions per second. Additionally, IOblend integrates seamlessly with leading cloud services like Snowflake and Microsoft Azure, underscoring its versatility and broad applicability in various data environments.
At its core, IOblend is an end-to-end enterprise data integration solution built with DataOps capability. It stands out as a versatile ETL product for building and managing data estates with high-grade data flows. The platform powers operational analytics and AI initiatives, drastically reducing the costs and development efforts associated with data projects and data science ventures. It’s engineered to connect to any source, perform in-memory transformations of streaming and batch data, and direct the results to any destination with minimal effort.
IOblend’s use cases are diverse and impactful. It streams live data from factories to automated forecasting models and channels data from IoT sensors to real-time monitoring applications, enabling automated decision-making based on live inputs and historical statistics. Additionally, it handles the movement of production-grade streaming and batch data to and from cloud data warehouses and lakes, powers data exchanges, and feeds applications with data that adheres to complex business rules and governance policies.
The platform comprises two core components: the IOblend Designer and the IOblend Engine. The IOblend Designer is a desktop GUI used for designing, building, and testing data pipeline DAGs, producing metadata that describes the data pipelines. The IOblend Engine, the heart of the system, converts this metadata into Spark streaming jobs executed on any Spark cluster. Available in Developer and Enterprise suites, IOblend supports both local and remote engine operations, catering to a wide range of development and operational needs. It also facilitates collaborative development and pipeline versioning, making it a robust tool for modern data management and analytics
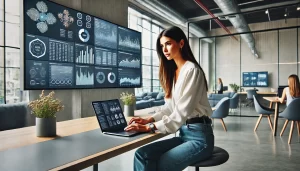
Optimising Customer Experience Through Real Time Data Sync
Optimising Customer Experiences Through Real Time Data Sync🧠 Fun Fact: Did you know that 90% of the world’s data has been created in just the past two years? That’s a lot of information to manage – and a massive opportunity for businesses that know how to use it wisely.Understanding your customers is the cornerstone of
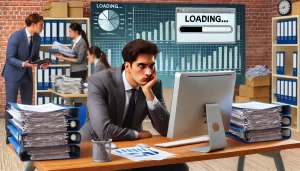
How Poor Data Integration Drains Productivity & Profits
How Poor Data Integration Drains Productivity & Profits Data is one of the most valuable assets a company can possess. We all know that (and if you still do not, god help you). Businesses rely on data to make informed decisions, optimise operations, drive customer engagement, etc. Data is everywhere and it’s waiting for us
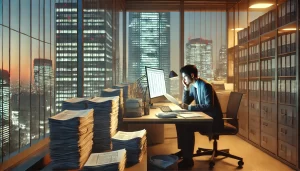
How To Unlock Better Data Analytics with AI Agents
How To Unlock Better Data Analytics with AI Agents The new year brings with it new use cases. The speed with which the data industry evolves is incredible. It seems that the LLMs only appeared on the wider scene just a year ago. But we already have a plethora of exciting applications for it across
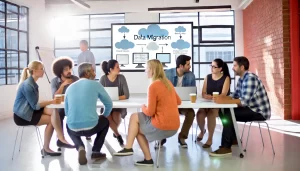
Why IOblend is Your Fast-Track to the Cloud
From Grounded to Clouded: Why IOblend is Your Fast-Track to the Cloud Today, we talk about data migration. Data migration these days mainly means moving to the cloud. Basically, if a business wants to drastically improve their data capabilities, they have to be on the cloud. Data migration is the mechanism that gets you there.
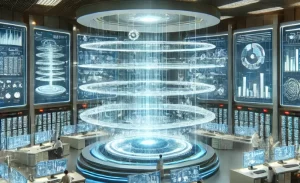
Data Integration Challenge: Can We Tame the Chaos?
The Triple Threats to Data Integration: High Costs, Long Timelines and Quality Pitfalls-can we tame the chaos? Businesses today work with a ton of data. As such, getting the sense of that data is more important than ever. Which then means, integrating it into a cohesive shape is a must. Data integration acts as a
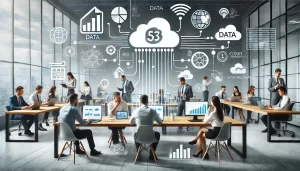
Tangled in the Data Web
Tangled in the Data Web Data is now one of the most valuable assets for companies across all industries, right up there with their biggest asset – people. Whether you’re in retail, healthcare, or financial services, the ability to analyse data effectively gives a competitive edge. You’d think making the most of data would have