The AI Hype Trap: Why Overblown Promises Backfire
AI hype has truly taken over the word of data. Every event you attend, everyone is discussing AI, ML, GenAI, LLMs, and AGI. Every data product seems to have some sort of AI features built into them. There are seemingly AI experts everywhere now. I am in the data industry and even I get lost in the flood of almost daily updates on new AI announcements. Lots of it is just noise. But if you shovel away the BS, you can indeed uncover some great gems for the analytics there.
Don’t get me wrong, I am a supporter. But a practical one. I hate the hype. I like to see tangible benefits of any tech before I become a believer. When we deploy IOblend, we expect it to make it a significant positive impact on the client’s challenges. We don’t want to sell them our solution if their current setup does the job just fine. Every new technology implementation brings with it changes to the BAU. Change tends to be disruptive. The benefits must be material enough to justify putting the company through the change process.
The AI implementation is no different. It must make a visible and material positive impact on the business or it’s a waste of money.
That’s not what’s yet happening though in many cases. Especially in the GenAI universe. The hype forces companies to think that they must deploy the tech wholesale or be forever left behind. Like a panacea for all their business problems. The businesses are expected to drop what they did with data and analytics already and throw resources at the AI. With gusto.
What’s happening then?
This is where the hyped-up promises of digital wonders meet the reality of technological limitations, business data and processes. The challenges often arise from the misplaced expectations by the senior management. “We gave you a big budget, why aren’t we seeing the impact yet?”
The biggest mistake here is thinking that AI is somehow different (i.e. simpler) from all the past technological breakthroughs and thus should be much quicker to implement and reap financial benefits.
It is not by any means.
Early adoption phase
Many businesses are still in the exploration phases, experimenting with AI (and the more recent GenAI) applications rather than implementing them at scale. The initial use cases often focus on innovation and proof-of-concept rather than revenue generation and cost reduction. AI is not easy. It is the cutting edge of technology and requires time to embed and gain wide adoption. Even the largest companies struggle with AI implementations (McDonald’s drive-thru AI pulled after multiple errors). And we’ve all seen the struggles Google is having.
Integrating AI into existing business processes and systems is complex, requiring significant investment in both time and resources. Just like any other technology companies always struggled to implement for decades now.
High initial costs
Adopting AI and GenAI solutions requires substantial upfront investment in technology infrastructure, such as high-performance computing resources and cloud services. A lot of businesses are still on-prem and buried in spreadsheets. Throwing AI at them wholesale brings a host of other expenses they might not be ready for or even anticipated.
Then, there is a high demand for skilled AI professionals, leading to increased costs for hiring, training and retaining talent.
Data dependency
Practical AI and GenAI models require large volumes of high-quality data to be effective. Many businesses struggle to gather, clean, and maintain the necessary data. That data problem is not AI-related but has plagued companies forever. The AI just put a bigger spotlight on it. Bad quality data makes the models produce spurious results, quickly diluting trust in the technology itself. “What is this rubbish? I’m not using it again.”
Concerns over data privacy and security regulations also limits the extent to which businesses can leverage GenAI in particular. Many businesses are very cautious about what data they share for training.
Regulatory and ethical concerns
The regulation for AI is still evolving, creating uncertainty for businesses about compliance requirements and potential legal risks. Moving goalposts.
On the ethical front, there are concerns around the use of AI, such as bias and transparency, can slow down the adoption process as businesses work to address these issues. Even small biases can have a significant impact on large-scale businesses, such as insurance brokers, mortgage lenders, etc.
Organisational readiness
Resistance to change can put massive obstacles to the adoption of new technologies. There are the obvious concerns around job security. “Will the AI replace what I do here?” The hype makes you believe that a lot of admin and entry level analytical jobs will be gone soon. Not the best way to win hearts and minds.
Then, if the employees start using the AI, they still need time to adapt to new workflows and tools. They need help training, handholding and close support by the AI experts (usually external consultants). Even for those who eagerly embrace the technology, there is still a learning curve. None of it happens overnight or comes at no cost.
Market dynamics
In some industries, there is a bit of “wait and see” approach happening among the senior leadership. The competitive advantages provided by AI and GenAI are not yet immediately apparent. At least not in a sufficiently material manner to make them go after the tech with full force. Especially in markets where competitors are also only just beginning to adopt the technology.
What we also observe is that many businesses didn’t grow their digital budgets to accommodate AI and GenAI. But rather they reallocated resources away from other digital activities. This shows the unwillingness to make big bets just yet.
And, naturally, broader economic conditions, such as market volatility, high cost of capital and geopolitical risks, impact business investment in new technologies when the payback is not immediately material.
Ignore the hype; it’ll save you money
So, what can the business do to avoid frustration when implementing AI and GenAI tech in production? Treat AI just as you would any other technological advancement. How long did it take your company to adopt data warehouses, ERP, CRM, or migrate to the cloud? How costly and painful was it? Revisit the learnings. AI will be no different.
Thoroughly evaluate implementation challenges
You have built your AI test models and demonstrated the positive effects on your business. Now it’s the time to scale out the PoC. Everyone is excited. Expectations run high.
First, choose the right use case, fully understanding the limitations of the AI technology. The hype will have you believing anything is possible. Do your research properly. Get a second opinion from an independent party before you commit to full deployment. It will save you a lot of money and potential embarrassment.
Then, you actually need to make it scale. This is when things become tricky. Putting a PoC into production often requires more time and effort that you have planned. Again, just like with any other technology.
One the biggest technical challenges that we encounter is issues with data. Mainly, it comes to the ability (or lack of thereof) to integrate, curate and manage vast amounts of data needed to feed the algorithms in production.
Data sources
First, the sheer volume and variety of data required for effective AI models requires robust data integration capabilities. Data coming from sensors, point solutions, events, databases/data lakes, batch and streaming must be aggregated and harmonised efficiently. This harmonisation leads to a unified data set that AI algorithms can then process to produce reliable outputs. And it must be done at scale and in an automated fashion to reduce errors and cost.
For instance, a financial institution deploying AI for fraud detection must integrate transaction data, customer profiles, and external threat intelligence. Without automated integration, this process would be laborious and error-prone, easily leading to significant gaps in the AI’s analysis.
Data quality
Once you automate the data collection, you need to ensure data quality and consistency. AI models are only as good as the data they are trained on. You need a consistent way to detect and rectify anomalies, fill missing values, and standardise data formats at scale. This continuous data curation is essential for the model’s performance and longevity. Doing this manually will never work at scale.
A case in point is the healthcare sector, where AI models assist in diagnosing diseases. The success of these models relies on the quality of medical records, imaging data, and patient histories. With automation of your data integration, they feed these models with the consistent, high-quality data, enhancing diagnostic accuracy and patient outcomes.
Real-time processing
Many operational uses cases with material benefits require dynamic decisioning. The AI in these settings will need a constant and reliable feed to robust data in real time.
For example, in autonomous driving, AI systems must process sensor data live to make split-second decisions. Real-time data integration and management make this decisioning possible by ensuring that data from various sensors is rapidly and accurately combined and analysed.
Take another example from the manufacturing industry where we helped bring automation of data integration. They had to process factory data manually once a week from seventeen plants. By the time they processed and analysed the data, events like stoppages, machine breakages, inventory levels had all been out of date. We moved them to real-time data processing and the analytics became live. It enabled them to start using AI for a more in-depth analysis.
If your business never worked with real-time data, that is another layer of technology that you need to adopt. Luckily, real-time data integration is a lot easier today than it used to be. Products like IOblend made that accessible and cost-effective. But that consideration must still be taken when deciding on AI investments.
ROI timeframe
Developing and deploying production-grade AI and GenAI solutions takes considerable time. The payback will not be immediate. A lot of the use cases now are compartmentalised PoCs and MVPs. The data is handpicked, manually curated and prepped. Scaling these out to production are lengthy and costly exercises, especially if the underlying data estates are not in tip-top conditions. Hint: most aren’t.
Measuring the direct financial impact of GenAI initiatives can also be challenging, especially in the early stages. ROI may be realised through indirect benefits like improved efficiency, customer satisfaction, and innovation rather than immediate revenue increases. Putting a $$ number on those benefits is not straightforward, so the CFOs tend to look at such investments with a degree of scepticism.
You can see in the recent market announcement that the AI-related stocks are starting to lose momentum. The market expectations were mismatched with the reality of the ROI timeframes. The hype had a lot to do with that.
Hype is never helpful
The AI hype is not helping the AI technology’s development and public perception. We all know why it’s done. There is a lot of money being invested in the industry. But this “overenthusiasm” leads to disillusionment and scepticism when the technology fails to deliver immediate, miraculous results. This then damages the genuine progress and investment.
The hype also obscures the realistic capabilities and potential limitations of AI, creating a gap between our understanding and actual technological advancements. The disconnect results in regulatory and ethical challenges. The policymakers and the public may not be adequately informed to address the real issues and potentials of AI, ultimately stalling its responsible and effective integration into society.
And finally, the hype pushes the businesses to rush implementations, which leads to expensive blunders. It’s critical to spend time understanding the technology and its implications on your business thoroughly beforehand. This requires some investment up front, but trust me, this is a lot cheaper than plunging vast resources into AI and seeing bubkis in return.
We, at IOblend, specialise purely in data integration, real-time and batch. We do full end-to-end data processing, management and technical governance before the data ever lands in the destination. This makes it an ideal tool to power AI applications automatically with curated data. Have a look at our use cases and the tech on our new website.
And, as always, we are always open for a chat.
IOblend presents a ground-breaking approach to IoT and data integration, revolutionizing the way businesses handle their data. It’s an all-in-one data integration accelerator, boasting real-time, production-grade, managed Apache Spark™ data pipelines that can be set up in mere minutes. This facilitates a massive acceleration in data migration projects, whether from on-prem to cloud or between clouds, thanks to its low code/no code development and automated data management and governance.
IOblend also simplifies the integration of streaming and batch data through Kappa architecture, significantly boosting the efficiency of operational analytics and MLOps. Its system enables the robust and cost-effective delivery of both centralized and federated data architectures, with low latency and massively parallelized data processing, capable of handling over 10 million transactions per second. Additionally, IOblend integrates seamlessly with leading cloud services like Snowflake and Microsoft Azure, underscoring its versatility and broad applicability in various data environments.
At its core, IOblend is an end-to-end enterprise data integration solution built with DataOps capability. It stands out as a versatile ETL product for building and managing data estates with high-grade data flows. The platform powers operational analytics and AI initiatives, drastically reducing the costs and development efforts associated with data projects and data science ventures. It’s engineered to connect to any source, perform in-memory transformations of streaming and batch data, and direct the results to any destination with minimal effort.
IOblend’s use cases are diverse and impactful. It streams live data from factories to automated forecasting models and channels data from IoT sensors to real-time monitoring applications, enabling automated decision-making based on live inputs and historical statistics. Additionally, it handles the movement of production-grade streaming and batch data to and from cloud data warehouses and lakes, powers data exchanges, and feeds applications with data that adheres to complex business rules and governance policies.
The platform comprises two core components: the IOblend Designer and the IOblend Engine. The IOblend Designer is a desktop GUI used for designing, building, and testing data pipeline DAGs, producing metadata that describes the data pipelines. The IOblend Engine, the heart of the system, converts this metadata into Spark streaming jobs executed on any Spark cluster. Available in Developer and Enterprise suites, IOblend supports both local and remote engine operations, catering to a wide range of development and operational needs. It also facilitates collaborative development and pipeline versioning, making it a robust tool for modern data management and analytics
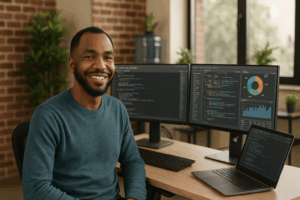
Rapid AI Implementation: Moving Beyond Proof of Concept
Rapid AI Implementation: Moving Beyond Proof of Concept 💻 Did you know that in 2024, the average time it took for a business to deploy an AI model from the experimental stage to full production was approximately six months? Bringing AI Experiments to Life The journey of an AI project typically begins with a “proof
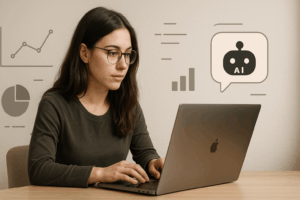
Agentic AI ETL: The Future of Data Integration
Agentic AI ETL: The Future of Data Integration 📓 Did you know? By 2025, the volume of data generated globally is projected to reach 175 zettabytes? That’s a truly enormous number, highlighting the ever-increasing importance of efficient data management. What is Agentic AI ETL? Agentic AI ETL represents a transformative evolution in data integration. Traditional
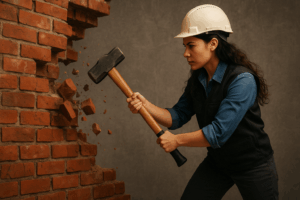
Break Down the Data Walls with IOblend
Break Down the Data Walls with IOblend 📑 Did you know? It’s estimated that a whopping 80% of business data is just floating about, unstructured and stuck in siloed systems. Siloed data only brings value (if at all!) to the domain it belongs to. But the true value lies in the insights in brings to
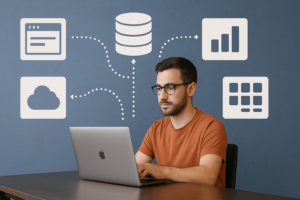
Put a Stop to Data Chaos with IOblend Governed Integration
Put a Stop to Data Chaos with IOblend Governed Integration 🤯💥Did you know? By 2025, the global datasphere is projected to grow to 175 zettabytes? This staggering figure underscores the sheer scale of data businesses must manage, making simplification not just a luxury, but a necessity. Today, businesses don’t have a shortage of data. What
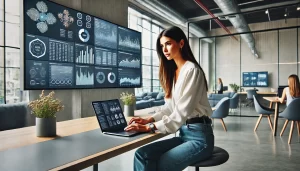
Optimising Customer Experience Through Real Time Data Sync
Optimising Customer Experiences Through Real Time Data Sync 🧠 Fun Fact: Did you know that 90% of the world’s data has been created in just the past two years? That’s a lot of information to manage – and a massive opportunity for businesses that know how to use it wisely. Understanding your customers is the
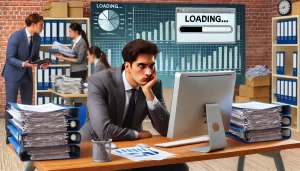
How Poor Data Integration Drains Productivity & Profits
How Poor Data Integration Drains Productivity & Profits Data is one of the most valuable assets a company can possess. We all know that (and if you still do not, god help you). Businesses rely on data to make informed decisions, optimise operations, drive customer engagement, etc. Data is everywhere and it’s waiting for us